Dear Brainstorm Team
I have been searching for quite a time but didn’t found an answer in the Form.
I want to calculate the Granger Causality (Spectral) on the Constrained Source of my Resting EEG.
If I want to calculate the Granger Causality on 4 Frequency bands, do I have to Calculate the Granger causality (Spectral) for each Frequency band ?
Or can I set the “Highest frequency of interested” to the highest Frequency I’m locking for.
And after that extract the Frequency bands with the “Group in Time or Frequency bands” Function?
Thanks for your Help
The "Grange causality (spectral)" will give you a spectrum similar to the one you obtain when using the PSD process: one value for each frequency bin of the FFT that is used in this process.
The option "Highest frequency of interest" only cuts all the values above a certain threshold after computation. It is more of storage and visualization option, it does not affect the values for the frequency bins below this maximum.
Calling the process "Group in frequency bands" after would average various frequency bins, meaning it would average various Granger scores together. I'm not sure this is a valid approach...
@hossein27en ?
1 Like
Thank you for taking the time to answer my Question and the well Explanation. Then I'm hoping that
hossein can help me there out.
@Francois
During my research about Connectivity on the Brainstorm Forum if found two Responses from you that are a bit Older and i have Questions about.
- In your Answer to the Question Functional connectivity measuring you said that the Option "after" in the Function “When to apply the scout function:" is the recommended approach.
Is this still the recommended Approach?
- In another Forum Entry Connectivity tool, you mentioned that in the Desikan-Killiany atlas, the regions are too large to be functionally homogeneous and averaging the source activity over large regions can cause that most of the interesting signals can be lost…
I totally agree with you that using in large Regions Interesting signals can be lost.
Do you have based on your Experience a guideline, what would be a good Size for Scouts? Or can you Recommand a Paper for that Issue?
Regards
Unfortunately, these are questions that I still can't answer better than in the threads you pointed at...
I'm hoping that the new connectivity documentation will address them.
@hossein27en?
2 Likes
To answer your former question, yes you can average among the values of Granger causality in different frequency bins.
For the next two questions regarding source connectivity:
As Francois said there are two approaches for that and generally it depends on your specific case that how to define scouts or whether first average sources and compute the connectivity measure or otherwise. Averaging among sources might either increase the SNR or cancel out signals depends on how you computed sources (PCA/Max/Mean).
There is also no optimal size for the scout. You should consider the anatomical region and surface/volume analysis.
@Francois and @hossein27en
Thank you both very much for your Help and taking the time to answer my Questions.
It's much clearer now and i'm nearly ready to start the Calculations.
During Preparing my Pipeline, one more Question come out.
Right now, i have for Each Subject around 100 Resting State (RS) Epochs with a duration from 1 Sec.
From this RS.
I used the RS to Calculate the Noise Matrix for the ERP(to provide evidence for a meaningful inverse solution)
After That I calculated the Constrained Source for all the RS Files.
For the Source Reconstruction i used the Diagonal noise covariance.
Now my Question is: Do i need to apply a baseline normalization on the Resting State Sources?
I would say No, because i would lose meaningful information but i'm not sure about that...
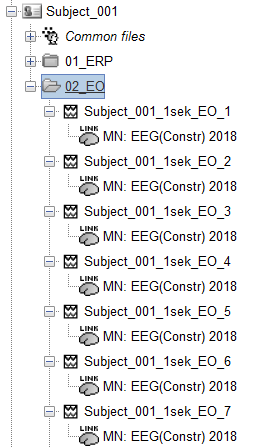
For the Source Reconstruction i used the Diagonal noise covariance.
Why didn't you use a ful noise covariance matrix?
If you compute it from all the recordings (ie. selecting all the files at the same time), you should have enough data available for a correct estimation of the covariance, no?
Do i need to apply a baseline normalization on the Resting State Sources?
Maybe yes, but it depends what you are expecting to do with these files (See the recommendations section):
https://neuroimage.usc.edu/brainstorm/Tutorials/SourceEstimation#Source_map_normalization
@Francois I used the Diagonal Matrix because in the Tutorial https://neuroimage.usc.edu/brainstorm/Tutorials/NoiseCovariance#Variations_on_how_to_estimate_sample_noise_covariance the said If the target brain activity to your experiment is resting you should calculate the noise over the Resting recordings (i used about 2 min of resting for that) and the only use the diagonal elements. Which i though i did by using the Diagonal noise covariance. while creating the source.
or am i on the wrong track?
And thanks for the hint with the recommendations section. Got my answer.
No this looks good, you read more carefully than I did 
1 Like